Difference between Artificial Intelligence and Machine Learning
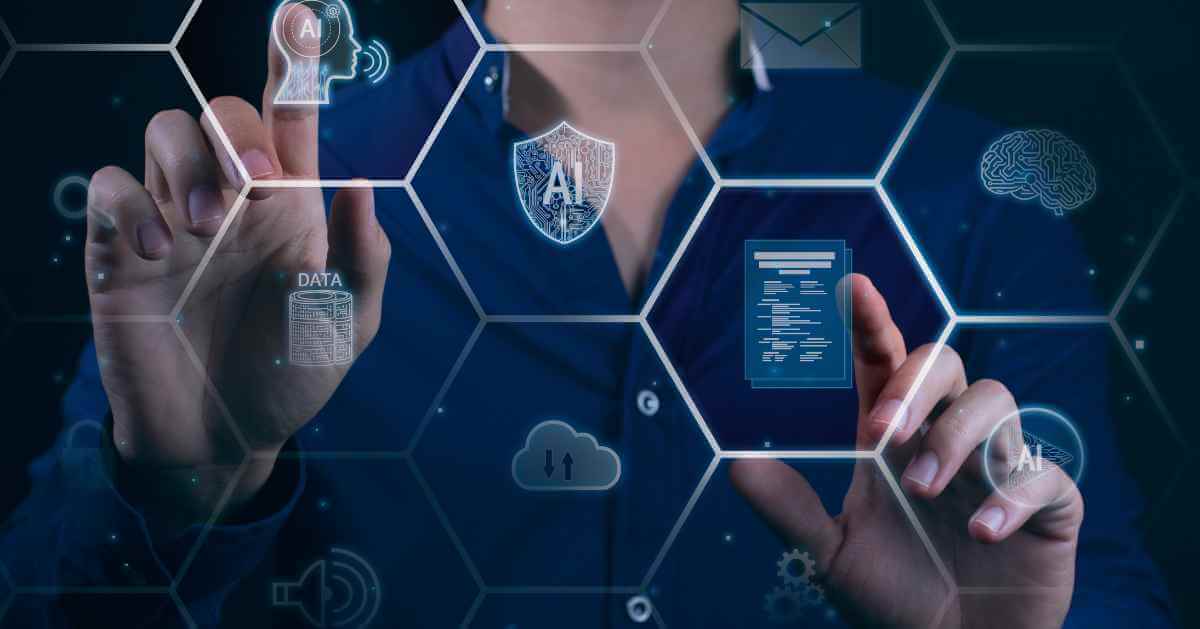
In the rapidly advancing landscape of technology, the terms “Artificial Intelligence” (AI) and “Machine Learning” (ML) are often used interchangeably, contributing to confusion about their distinctions. While both are integral to computer science, they represent distinct domains with unique functionalities. Understanding the nuances between Artificial Intelligence and Machine Learning is essential for navigating the complex landscape of intelligent technologies. In this blog, we embark on a journey to unravel the intricacies of AI and ML, shedding light on their definitions, functionalities, and the pivotal role each plays in shaping the future of technology. Join us as we demystify the boundaries between these two transformative fields and explore how they collaborate to redefine the possibilities of computational intelligence.
Thank you for reading this post, don't forget to subscribe!A career in the Artificial Intelligence (AI) and Machine Learning (ML) landscape is exceptionally dynamic and promising. As industries increasingly leverage AI and ML technologies, professionals find themselves at the forefront of innovation. The demand for skilled AI and ML practitioners spans diverse sectors, including healthcare, finance, technology, and more. Roles vary from machine learning engineers and data scientists to AI researchers and solution architects.
A PG in AI and ML significantly boosts career prospects by offering in-depth knowledge, hands-on experience, and specialization in key areas. These programs provide industry-relevant skills aligned with current demands, making graduates highly competitive. Practical projects enhance problem-solving abilities, and networking opportunities connect students with professionals and potential mentors. Access to cutting-edge resources and industry connections ensures staying abreast of the latest advancements.
A Postgraduate (PG) program in Artificial Intelligence (AI) and Machine Learning (ML) significantly boosts career prospects by offering in-depth knowledge, hands-on experience, and specialization in key areas. These programs provide industry-relevant skills aligned with current demands, making graduates highly competitive. Practical projects enhance problem-solving abilities, and networking opportunities connect students with professionals and potential mentors. Access to cutting-edge resources and industry connections ensures staying abreast of the latest advancements.
What is Artificial Intelligence?
Artificial Intelligence involves creating computers and robots that mimic and surpass human capabilities, analyzing data to provide information or automate actions. It powers technologies like voice assistants and smart devices, using natural language processing and computer vision to automate tasks, expedite decision-making, and facilitate customer interactions with chatbots. Today, AI is integral to various technologies, shaping how we interact with devices and automating processes across industries.
What is Machine Learning?
Machine learning, a sub-domain of artificial intelligence, employs algorithms to autonomously learn from data, enhancing decision-making by recognizing patterns. Programmers, exploring the potential of machine learning, push the boundaries of improving a computer system’s perception, cognition, and action. Deep learning is an advanced form of machine learning, takes this further by utilizing large neural networks resembling the human brain. These networks analyze data logically, enabling learning intricate patterns and making predictions autonomously without human intervention.
Key differences: Artificial Intelligence vs. machine learning
Machine learning (ML) operates as a distinct subset of artificial intelligence (AI), showcasing a more limited scope and focus than the broader field of AI. While ML concentrates on specific techniques for pattern recognition and learning from data, AI encompasses a wider array of strategies and technologies that extend beyond the purview of machine learning.
Here are some key differences between Artificial Intelligence and Machine Learning:
Objectives
The primary aim of any AI system is to efficiently execute complex human tasks, encompassing learning, problem-solving, and pattern recognition. Conversely, the goal of machine learning (ML) is centered on having a machine scrutinize substantial datasets. Employing statistical models, the machine identifies patterns within the data, generating results with a probability of correctness or a degree of confidence.
Methods
In AI, numerous methods are employed to address a spectrum of challenges. This toolkit encompasses genetic algorithms, neural networks, deep learning, search algorithms, rule-based systems, and the overarching field of machine learning.
Within machine learning (ML), methods are categorized into two primary types: supervised and unsupervised. Supervised ML algorithms tackle problems by learning from input and output data values. In contrast, unsupervised learning takes an exploratory approach, seeking to unveil concealed patterns within unlabeled datasets.
Types
Artificial Intelligence (AI) encompasses various types, with Machine Learning (ML) being a prominent subset. AI can be categorized into three main types: Narrow or Weak AI, General or Strong AI, and Artificial Superintelligence. Narrow AI refers to systems designed for a specific task, like virtual personal assistants. General AI, still theoretical, would possess human-like cognitive abilities and adaptability across diverse tasks. Artificial Superintelligence is a hypothetical stage where AI surpasses human intelligence comprehensively.
Machine Learning, a subset of AI, has two primary types: Supervised Learning and Unsupervised Learning. Supervised Learning involves training models on labeled datasets, with input-output pairs guiding algorithm behavior. Unsupervised Learning lacks labeled outputs, encouraging algorithms to identify patterns and relationships independently within the data. Additionally, there’s Semi-Supervised Learning, Reinforcement Learning, and more, each serving specific AI and ML applications, fostering innovation across industries by addressing diverse problem domains and learning scenarios.
Implementation
Artificial Intelligence (AI) and Machine Learning (ML) implications are profound and far-reaching. On the positive side, AI and ML holds potential to revolutionize industries, enhance efficiency, and solve complex problems. In healthcare, they can aid in early disease detection and personalized treatments. In finance, they optimize trading strategies and risk management. However, concerns arise regarding job displacement due to automation, ethical considerations in decision-making algorithms, and the potential for biased outcomes. Security and privacy issues also surface with the increasing reliance on intelligent systems. Therefore, striking a balance between innovation and responsible implementation becomes crucial to harness the benefits of AI and ML while mitigating potential risks, ensuring a future where technology aligns with societal well-being and ethical standards.
Conclusion
In conclusion, discerning the difference between Artificial Intelligence (AI) and Machine Learning (ML) is foundational to understanding the intricacies of the evolving technological landscape. Pursuing a PG in AI and ML becomes pivotal, offering comprehensive insights into these domains and preparing individuals for impactful careers. As AI and ML continue to shape industries, a nuanced understanding becomes a professional asset and a prerequisite for navigating ethical considerations. A PG program equips aspirants with the knowledge and skills needed to contribute to this dynamic field, ensuring a meaningful and successful career in the fast-growing domain of AI and ML.